The Importance of Data Labeling in Machine Learning for Modern Businesses
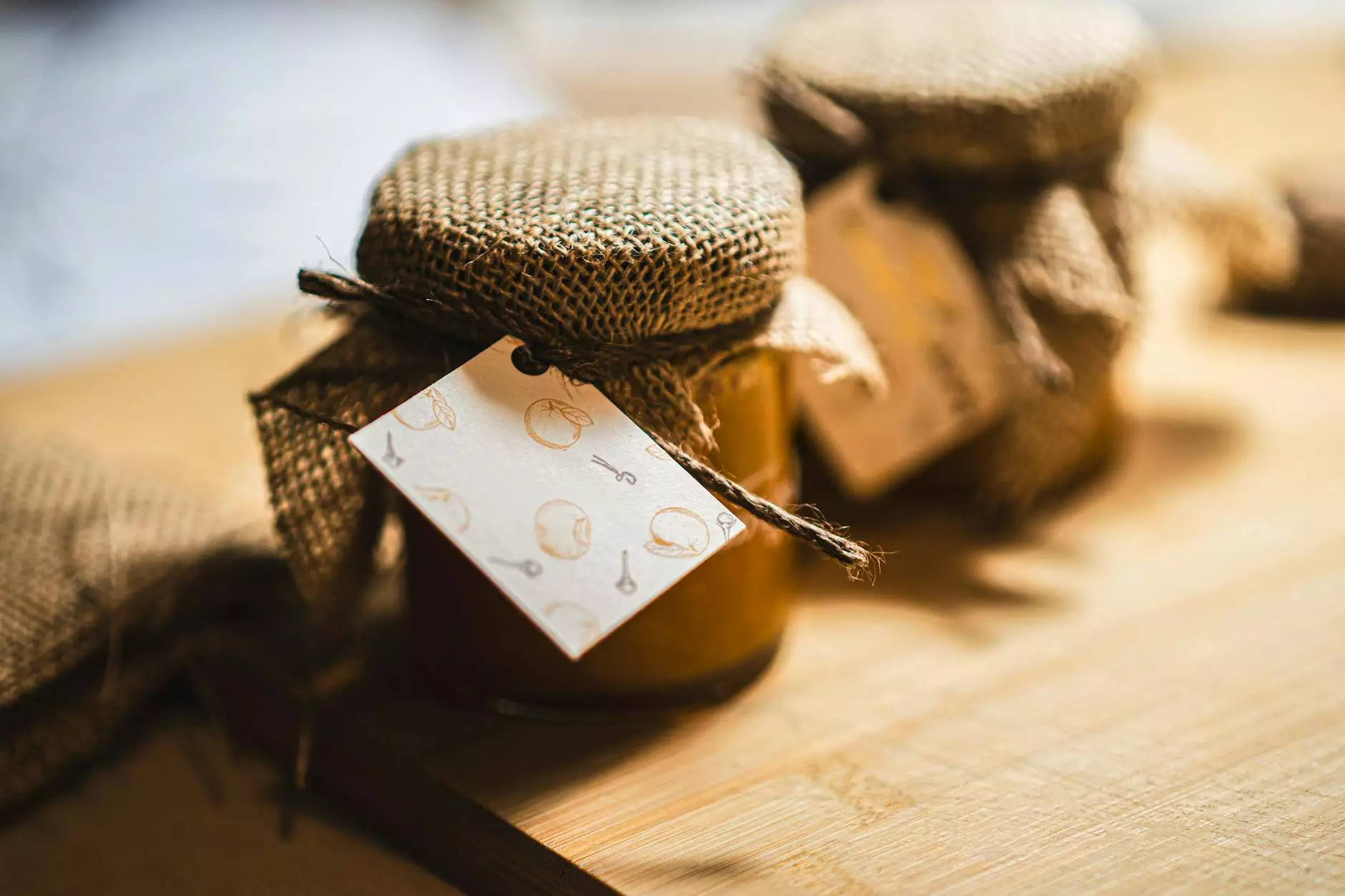
In today’s digital era, businesses rely heavily on data labeling and machine learning techniques to drive innovation, enhance decision-making, and deliver exceptional customer experiences. This article delves deep into how data labeling acts as the foundation of machine learning, helping companies like Keymakr leverage their data for transformative outcomes.
Understanding Data Labeling
Data labeling is the process of annotating or tagging datasets to make them usable for machine learning algorithms. It involves identifying and categorizing data elements, making it easier for machines to learn from and make predictions. This crucial step is not just about adding labels but is pivotal to the success of any machine learning model. Here are some key points to understand data labeling:
- Definition: Data labeling is the process of assigning meaningful labels to data points in datasets.
- Types: It can include image annotation, text classification, audio transcription, and more.
- Methods: Data labeling can be done manually by human annotators or automatically through algorithms.
- Tools: Many software tools and platforms facilitate efficient data labeling processes.
Why is Data Labeling Crucial for Machine Learning?
Machine learning systems learn patterns based on the data they are trained on. Without properly labeled data, these systems could misinterpret the information, leading to inaccurate predictions and analyses. Here’s why data labeling is instrumental:
1. Enhances Model Accuracy
Accurate data labeling ensures that the machine learning model learns the correct relationships in the data. When labels are correctly assigned, models can achieve higher accuracy, which ultimately leads to improved decision-making for businesses. This leads to better outcomes in areas such as:
- Customer Insights: Understanding customer behavior better through data-driven decisions.
- Predictive Analytics: Accurately forecasting trends and customer needs
2. Facilitates Training of Supervised Learning Models
In supervised learning, models learn from labeled datasets. Without correct labels, the training process becomes erratic and prone to errors. The importance of data labeling in this context includes:
- Model training: Helping models recognize features and improve performance over time.
- Performance Measurement: Establishing baseline metrics to evaluate model efficacy.
3. Supports Diverse Applications
Data labeling allows for a wide range of applications across various industries. For instance:
- Healthcare: Labeling medical images to aid in cancer detection.
- Finance: Classifying transactions for fraud detection.
- Retail: Analyzing customer feedback for product improvement.
The Process of Data Labeling
The data labeling process can be expansive, intricate, and varies based on project requirements. Here’s how businesses typically approach data labeling:
Step 1: Define the Objective
Before labeling begins, it is essential to clearly define the objective of the machine learning model. What problem is it attempting to solve? Understanding the end goal helps in determining the labeling requirements.
Step 2: Data Collection
Once the objectives are set, businesses need to gather relevant data that fits their criteria. This data can come from various sources such as:
- Public datasets: Open-source data available from research and governmental organizations.
- Internal databases: In-house data collected through customer interactions.
Step 3: Select Labeling Method
Next, businesses must choose a labeling method. They can opt for:
- Manual Labeling: Engaging human annotators to label data manually.
- Automated Labeling: Utilizing machine learning tools to automate the labeling process.
Step 4: Conduct Quality Assurance
Quality assurance is vital in the data labeling process. Companies should establish verification steps to ensure labels are accurate, consistent, and free from bias. Continual review and adequate training for annotators can greatly enhance quality.
Step 5: Finalize the Dataset
Once the labeling is complete and quality checks have been conducted, the final dataset is prepared for use in training machine learning models.
Challenges in Data Labeling
Despite its importance, data labeling comes with its own set of challenges. Businesses should be aware of these hurdles:
1. Scale
As the amount of data continues to grow exponentially, the need for large-scale labeling becomes a daunting task. Businesses must find efficient ways to handle big data while maintaining quality standards.
2. Cost
Data labeling can be a costly affair, particularly if manual labeling is required. Companies need to balance budget constraints while ensuring high-quality data labeling.
3. Subjectivity
Human annotators may hold biases, leading to inconsistencies in data labeling. Ensuring diverse teams and robust training can help mitigate this issue.
Future of Data Labeling in Machine Learning
The future of data labeling and its vital role in machine learning appears bright as advancements in technology continue to unfold. Here are some trends to watch out for:
1. Automation and AI Innovations
With the rise of artificial intelligence, automated labeling solutions are becoming more prevalent. These technologies can assist in speeding up the labeling process and improving accuracy over time.
2. Advanced Quality Control Measures
As businesses strive for higher-quality datasets, innovative tools that promise advanced quality control measures are on the rise. Expect remarkable developments in how businesses can maintain labeling accuracy.
3. Integration of Machine Learning in Labeling
Machine learning itself is being leveraged for labeling purposes, where models can learn to label data autonomously, reducing the need for human effort.
Choosing a Data Labeling Partner
Selecting the right partner for data labeling can significantly impact the success of your machine learning initiatives. Factors to consider include:
- Experience: Look for companies specializing in machine learning and data labeling.
- Capabilities: Ensure they can handle your specific labeling needs at scale.
- Quality Assurance Processes: Inquire about their protocols for maintaining consistent quality.
Conclusion
In conclusion, data labeling is an essential component of machine learning that allows businesses to harness the full potential of their data. Companies like Keymakr demonstrate the importance of effective data labeling strategies in developing successful machine learning applications. As we move towards a more data-driven future, embracing efficient data labeling practices will undoubtedly help businesses achieve a competitive edge in their respective industries.
Investing in quality data labeling is not just a technical requirement; it embodies a strategic approach to leveraging insights that can revolutionize how organizations operate and engage with their customers. Embrace the power of data labeling in machine learning and watch your business thrive.
data labeling machine learning