Unlocking the Power of Labeling Tools for Machine Learning
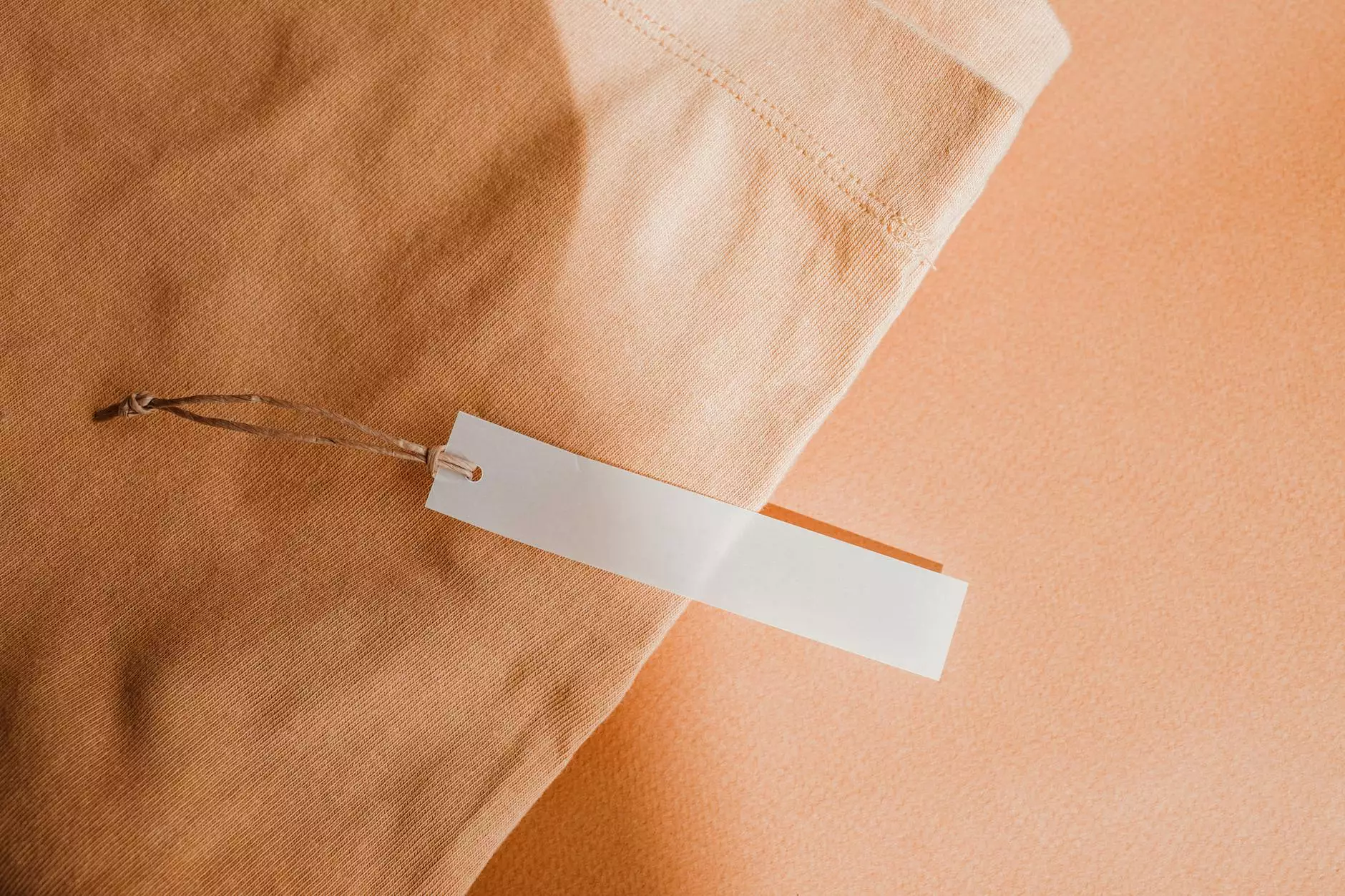
In an era where data is the new oil, labeling tools for machine learning stand as the unsung heroes that convert raw data into valuable insights. As organizations embark on their machine learning journeys, understanding the significance of data annotation becomes paramount. This article delves into the world of labeling tools, elucidating their importance, benefits, and how platforms like KeyLabs AI are redefining the standards of data annotation.
The Importance of Labeling Tools in Machine Learning
Machine learning algorithms thrive on large quantities of data. However, not all data is created equal; in fact, much of it requires careful labeling to be effective. Labeling tools for machine learning serve as the bridge between unstructured data and actionable intelligence. Here are key reasons why these tools are crucial:
- Data Quality: Properly labeled data ensures machine learning models are trained on accurate information, leading to better predictions.
- Model Accuracy: The fidelity of machine learning models relies heavily on high-quality annotations, which determine their performance.
- Scalability: Automated labeling tools can scale up the annotation process, accommodating large datasets swiftly.
- Efficiency: Advanced tools streamline the workflow, reducing the time and effort needed for data preparation.
Types of Labeling Tools Available
Not all labeling tools are the same. Depending on your specific project requirements, choosing the right type of labeling tool can make a significant difference. Here are some of the popular categories of labeling tools for machine learning:
1. Video Annotation Tools
Video annotation tools are designed for projects that require the analysis of visual media. Whether you are working on autonomous vehicle technology or surveillance systems, these tools allow you to tag and categorize objects within video frames. Key features typically include:
- Frame-by-Frame Annotation: Labeling objects in each frame independently for greater accuracy.
- Object Tracking: Enables consistent labeling of objects as they move throughout a video.
2. Image Annotation Tools
Image annotation involves labeling images for various machine learning tasks, such as image classification or object detection. Common methods include:
- Bounding Boxes: Drawing boxes around objects to identify their locations.
- Polygons: More complex forms of labeling that allow for more accurate delineation of object shapes.
- Semantic Segmentation: This involves labeling each pixel in an image to define the object boundaries precisely.
3. Text Annotation Tools
Text annotation tools play a crucial role in natural language processing (NLP) projects. They allow for the classification and labeling of text data, which is essential for tasks like sentiment analysis or entity recognition. Text annotation features often include:
- Named Entity Recognition: Identifying and categorizing key entities in text.
- Part-of-Speech Tagging: Labeling the grammatical parts of each word to understand its function in sentences.
Choosing the Right Labeling Tool for Your Needs
Selecting the appropriate labeling tools for machine learning projects can be daunting with so many options available. Here are some factors to consider when making your decision:
1. Project Requirements
Your specific machine learning project will dictate the type of data you are working with. Understanding the fundamental requirements of your project will help you choose the most effective tool.
2. User Interface
An intuitive user interface can drastically improve efficiency. Prospective users should look for tools that are user-friendly and easy to navigate, allowing team members to onboard quickly.
3. Customization and Flexibility
The ability to customize labeling tools to suit unique project needs can provide additional value. Look for tools that offer adaptability in labeling workflows and formats.
4. Integration Capabilities
How easily can the labeling tool integrate into your existing data infrastructure? Integration with other data processing tools or platforms can streamline the overall project workflow.
The Role of KeyLabs AI in Data Annotation
At KeyLabs AI, we pride ourselves on offering top-tier data annotation solutions tailored for various industries. Our innovative labeling tools for machine learning are designed to enhance the efficiency and accuracy of data preparation processes. Here’s how our platform stands out:
1. Comprehensive Annotation Solutions
Our platform provides tools for annotating images, videos, and text. No matter the data type, we have the resources to facilitate accurate labeling, ensuring your machine learning models are built on a solid foundation.
2. AI-Powered Automation
Our labeling tools for machine learning utilize cutting-edge artificial intelligence to enhance the efficiency of the annotation process. By automating repetitive tasks, we help you save time and reduce costs.
3. Collaboration and Scalability
KeyLabs AI is designed for teams of all sizes. Our platform allows for seamless collaboration among team members, ensuring that everyone can contribute to the data annotation process simultaneously. This collaborative approach can significantly speed up project timelines.
4. Quality Assurance
Quality is at the core of what we do. Our tools come equipped with features that allow for rigorous quality checks, ensuring that every piece of data is meticulously labeled before use in machine learning models.
Challenges in Data Annotation and How to Overcome Them
While labeling tools are incredibly valuable, the data annotation process is not without its challenges. Here are common hurdles encountered in data annotation and how to address them:
1. Time Constraints
Large datasets can require significant time commitments for annotation. Utilizing automated labeling tools, like those found in the KeyLabs AI suite, can help mitigate this challenge by speeding up the process.
2. Maintaining Consistency
Ensuring that all labeling is consistent across various annotators can be difficult. Setting clear guidelines and utilizing collaborative tools can help maintain quality and uniformity across all data annotations.
3. Cost Management
Data annotation can be resource-intensive. Organizations can optimize costs by leveraging automated solutions that reduce the need for extensive manual labor while still achieving high-quality results.
Future Trends in Labeling Tools for Machine Learning
As machine learning continues to evolve, so does the landscape of data annotation. Here are some trends to watch for in the future:
1. Increased Automation
Future labeling tools will increasingly utilize AI to assist in annotation, further reducing manual input and enhancing efficiency.
2. Enhanced Collaboration Features
Collaboration will become more integrated in labeling tools, enabling teams to work more effectively together, regardless of their geographic locations.
3. Focus on Unsupervised Learning
As unsupervised learning gains traction, labeling tools will adapt to support these methodologies, allowing for advanced data processing without extensive labeled datasets.
Conclusion
In summary, the importance of labeling tools for machine learning cannot be overstated. Organizations aiming to harness the full potential of their data must invest in high-quality data annotation tools to ensure machine learning models deliver accurate and reliable predictions. With platforms like KeyLabs AI, businesses can simplify their data annotation processes, optimize costs, and maintain the quality of their labeled data. Embrace the power of advanced labeling tools and propel your machine learning initiatives to new heights!